What is Prescriptive Analytics? Exploring Key Terms, Use Cases and Drivers
Organizations are racing to improve the pace and quality of decision-making. To do this, many are relying on prescriptive analytics. Prescriptive analytics is a type of advanced analytics that optimizes decision-making by providing a recommended action. It’s not uncommon for people to ask how prescriptive analytics differs from other analytics types or AI categories. Let’s unpack what prescriptive analytics means, explore some use cases, and look at what’s driving adoption.
What is Prescriptive Analytics?
Prescriptive analytics is often called the “final frontier” of analytics. Unlike descriptive (focused on reporting with basic trend or pattern recognition) or predictive analytics (focused on predicting the future), prescriptive analytics determines ways in which business processes should evolve or be modified. It provides implementable solutions with known costs and/or benefits. In other words, it makes data and business rules actionable instead of only providing insight into your data’s behavior.
As Gartner analyst Noha Tohamy explains in Hype Cycle for Supply Chain Strategy, 2021, “through prescriptive analytics, an organization can find strategies to meet its objectives by balancing trade-offs among conflicting goals.”
Prescriptive Analytics, Mathematical Optimization, and AI
Terms like prescriptive analytics, artificial intelligence (AI), and mathematical optimization are often used interchangeably. While they are closely related, they don’t mean exactly the same thing.
Mathematical optimization, and specifically optimization modeling, is about describing a business problem in mathematical formulas or equations. It symbolically describes the decisions you need to make, the restrictions you need to uphold, and the relations between the two, in order to achieve a given objective.
Mathematical optimization is often seen as “the leading prescriptive analytics tool in the market.” In other words, mathematical optimization is a type of prescriptive analytics. Want to learn more about prescriptive analytics? Download our Guide to Mathematical Optimization.
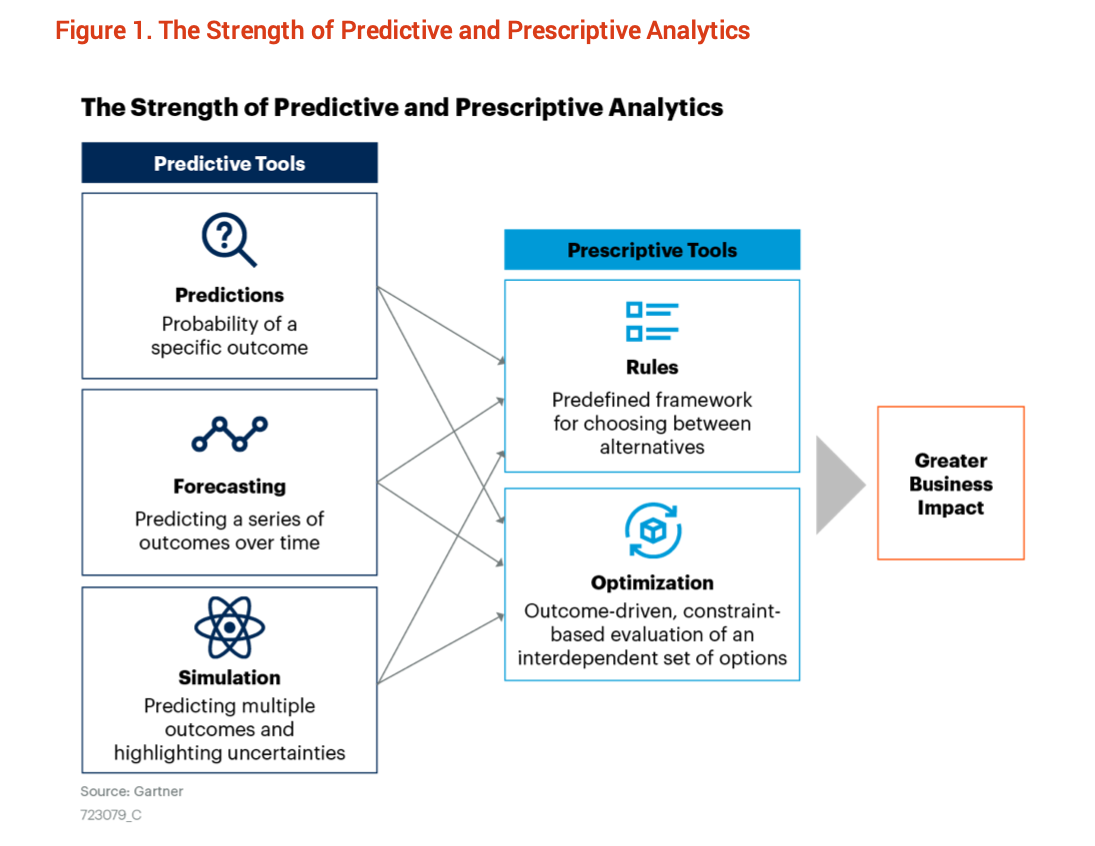
Artificial Intelligence is when machines that are programmed to think and learn like humans. It is a multidisciplinary field of computer science that aims to create systems capable of performing tasks that typically require human intelligence, such as learning, reasoning, problem-solving, perception, and interaction.
Gartner defines AI as, “a computer engineering discipline that uses a series of mathematically or logic-based techniques that uncover and capture coding knowledge and leverage sophisticated and clever mechanisms to solve problems to interpret events, support and automate decisions, and take actions.”
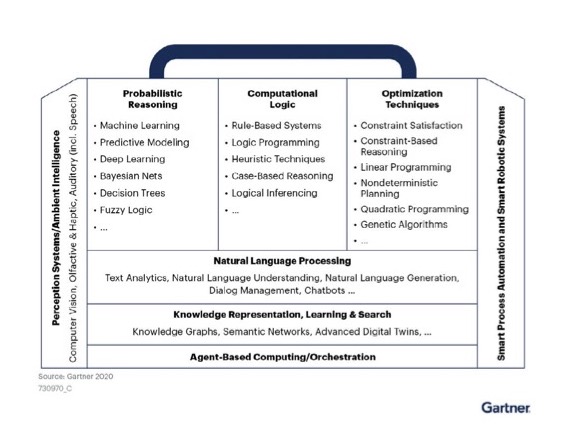
Although the hype around AI is fairly recent, AI techniques like mathematical optimization have been around for several decades.
Prescriptive Analytics use cases
Prescriptive analytics has been used since the 1980s in industries that focus on resource extraction, like the energy business. However, the use of this type of analytics is expanding across sectors. According to Allied Market Research, the prescriptive analytics market size was valued at $1,968.4 million in 2018 and is projected to reach $12.35 billion by 2026, growing at a CAGR of 26.6% from 2019 to 2026.
The use of mathematical optimization techniques is also growing in markets where it has not been as widespread. Brazil-based Volnei dos Santos, Technical Director at UniSoma (an analytics consulting firm), states, “until the beginning of the last decade, most of our projects focused on steel, pulp and paper, and animal production businesses. We started seeing a broader range of industries using optimization in the last ten years, like manufacturing, beverages, education, and more. Diversification has been the theme of the last decade.”
Prescriptive Analytics can be applied to optimize strategic, tactical, and operational decisions.
- A common use case is supporting supply chain network design decisions, such as where to invest in depot locations, optimizing for costs, or rationalizing a network after a merger.
- Other use cases include – capacity planning, energy dispatch planning, material blending, inventory and replenishment planning, sales and operations planning, and logistics optimization.
See how companies across industries use prescriptive analytics platforms like AIMMS.
Prescriptive Analytics tools
As we mentioned above, prescriptive analytics tools like mathematical optimization have a long and established history. Mathematical modeling languages like AIMMS emerged in the 80s. These languages formulated a standard way to define and solve prescriptive analytics models (using variables, constraints, and objectives). They reduced the need to code models from the ground up and offered the ability to interface with different mathematical solvers, which are algorithmic engines used to solve the mathematical problem at hand.
In recent years, we have seen the emergence of platforms. Prescriptive Analytics platforms, as defined by Gartner, “primarily focus on creating optimization solutions,” providing “data prep, prescriptive model building, model management and model deployment in various business processes.”
Traditionally, prescriptive analytics solutions have been built by experts, and in many cases, organizations prefer to build bespoke applications for very specific business needs. AIMMS provides a low-code platform that enables you to develop and deploy bespoke optimization applications for end-users, using a variety of mathematical programming types in the backend. On average, AIMMS customers are able to develop and deploy mathematical models 10-20 times faster.
Over time, we also noticed a growing market for off-the-shelf solutions. This led us to launch applications like SC Navigator and AIMMS IBP. The capability companies choose can be insourced, outsourced, or a hybrid of both, depending on the team’s skillset and the problem at hand.
What’s driving Prescriptive Analytics adoption?
Many factors are driving the adoption of these types of prescriptive analytics solutions.
1. Better decisions in complex environments
One of the primary drivers for prescriptive analytics adoption is the sheer complexity of today’s supply chains. “Business users face millions of possible choices when taking action” and “supply chains have become more distributed, spanning functions, regions and extending to external trading partners.” As Noha Tohamy explains, this often makes it impossible for humans to identify the best course of action to meet their goals.
2. Data availability, computing power, and ease of deployment
The increase in data availability and advances in computing power have also facilitated adoption in recent years. Cloud deployment has become the norm as well. The shift to the cloud significantly “un-ITd” the consumption of optimization and opened a world of possibilities when it comes to scalability. While in the past, organizations were dependent on the prioritization, capacity, and skills of their company’s IT departments to deploy optimization models, today they can do it within a few clicks. This makes it easier to operationalize this type of initiative.
3. A focus on operationalization
AIMMS partner Frans van Helden from ORTEC recalls, “Back in the day, the application of mathematics was known as OR. These days, it’s called data science and it’s gone from a niche practice to a must-have capability.” Pilot projects have run their course. Management teams know what prescriptive analytics is and they recognize that they can gain value from it. Many are shifting from pilots to operationalization. Gartner estimates that “by the end of 2024, 75% of enterprises will shift from piloting to operationalizing AI, driving a 5X increase in streaming data and analytics infrastructures.”
4. The ROI of prescriptive analytics
Prescriptive analytics models have considerable impact and proven ROI. Manufacturers using prescriptive analytics models for material blending typically see cost reductions of 3% or more, and logistics optimization models can deliver over 30% in savings. Vehicle loading optimization models also help trucks drive fewer miles, which results in a lower carbon footprint.
Recently, one of our manufacturing customers identified $20 million in savings during the first week of their modeling project. Their new integrated planning model, developed in AIMMS, showed that they could use their existing network capacity more effectively instead of investing in more (in this specific case an extra production line).
Is your organization ready to leverage Prescriptive Analytics?
The journey toward prescriptive analytics in your supply chain may require effort, but the rewards are substantial. From demand forecasting and inventory management to route optimization and real-time decision-making, prescriptive analytics empowers you to make smarter, data-driven choices that can lead to a competitive edge in today’s dynamic business landscape. You might want to explore 5 powerful prescriptive analytics examples in the supply chain.